This post has already been read 8908 times!
How generative AI is poised to disrupt project delivery and accelerate and amplify business value for manufacturers
In recent years, the industrial manufacturing sector has encountered significant upheavals due to challenges arising from geopolitics, rapid technological advancements, and the pandemic. These factors have prompted substantial shifts in how manufacturers conceive, execute, and deliver their products and services.
According to the Standish Group, over $48 trillion is spent annually on the delivery of new initiatives. On average, only approximately 30% of these initiatives are successfully delivered, meaning they are completed on time and within budget. The imperative to develop intelligent, interconnected products, digitalize operations, streamline supply chains through automation, and meet increasingly demanding customer expectations has created significant opportunities for radical growth.
“Artificial Intelligence is the new electricity. It is revolutionizing manufacturing by transforming production lines into smart factories, increasing productivity, driving innovation, and creating new business models.”
Andrew Ng, Adjunct Professor at Stanford University
However, many manufacturers’ existing methodologies, characterized by fragmented teams, function-centric approaches over strategic thinking, and static perceptions of competitive and operational landscapes, pose substantial risks to their ability to reinvent themselves effectively. Legacy manufacturers striving to embrace the future of smart products, digital platforms, and service-oriented business models urgently require a more effective path forward.
An innovative, hybrid approach that nurtures future ventures while concurrently enhancing current products and operations offers practical, value-driven solutions both in the short and long term.
Let’s examine some areas that manufacturers can leverage generative AI and advanced technology to improve their operational efficiency and maximize value delivery.
Digital Twins to Predict and Perfect
Digital twins refer to the creation of digital replicas of current or future manufacturing assets, operations, or even entire supply chains that can simulate all the characteristics of their physical counterparts. This is achieved by creating a series of digital models that leverage multiple processing sources to develop an accurate representation of a physical object, system, or process.
Today, more than 75% of advanced industries have adopted digital twin technologies, and that number will continue to grow since it offers a risk-free environment for the development of new designs, eliminating the expenses associated with producing and testing physical prototypes.
How AI Can Maximize Value in Project Delivery in Advanced Manufacturing Share on XIn other words, organizations can build and test before actually having the capital asset on hand. This means they can make adjustments and fine-tune it before investing in actually making the physical asset. Digital twins also enhance testing and validation processes by enabling the evaluation of new solutions across a wide range of scenarios, including unconventional and extreme operating conditions. Such testing enhances the product’s resilience in extreme and rare conditions.
Finally, digital twin technology helps predict the most effective product enhancements by simulating the effects of proposed design alterations using information gathered from products in operation. Systems can now be pieced together to near-perfection instead of making large upfront investments that may lead to technical inefficiencies and operating waste.
Machine Learning to Improve Delivery
Project Management has been a science for years. Over the course of the last decade, there has been a strong push towards applying project-specific methodologies that increase the success of a transformation projects.
Whether waterfall, agile, hybrid, or systems development life cycle (SDLC), each methodology has its unique way of grouping and structuring the work to better organize and facilitate execution.
However, successful transformation often depends on years of experience gathered from the field. Even experienced professionals often use past examples or industry best practices to drive implementations, but overlook project-specific requirements that can undermine the success of the entire project. Their information sources are often ad hoc, scattered, and/or dependent on other people making the correct decisions.
"AI-driven project scheduling utilizes data from previous projects to generate a range of potential schedules based on specific project attributes, then proactively alerts managers to potential risks to the timeline and project." -Sokratis Vasilas Share on XTo fix some of these issues, early AI machine learning models were introduced, offering insights to project managers regarding optimal project setup and direction based on specific parameters. They help respond to issues and risks, drawing from successful strategies employed in past to drive favorable outcomes in the current project.
While there are only a handful of instances where machine learning has been effectively integrated into transformation delivery, some examples include:
- Modifying scheduling components based on user permissions and preferences
- Using social tagging to identify and connect users for tasks
- Using machine learning-based project analytics to predict metrics such as expected net promoter score (NPS) and client satisfaction
For example, AI-driven project scheduling could incorporate insights gleaned from past projects and propose a range of potential schedules based on similar project requirements, attributes, and metadata based on past projects.
Additionally, project plans could be dynamically adjusted and re-baselined in nearly real-time, based on historical team performance and project advancements. Then the AI system could proactively notify project managers of potential risks and opportunities through real-time analysis of project data.
Predictive Quality & Maintenance
AI could also make a significant impact in improving quality and maintenance. Up until today, there has been a traditional approach taken when it comes to maintaining the physical assets of manufacturing operators that are ultimately the ones to produce the required products.
Approach 1 is reactive maintenance, which entails repairing equipment solely upon breakdown. Despite appearing economically efficient at first glance, this strategy can result in prolonged periods of inactivity, escalated repair expenditures, and diminished overall efficiency.
Approach 2 is preventative maintenance, which relies on predetermined interventions, like regular inspections or replacements, aimed at averting equipment failures. Although this approach decreases the chances of unforeseen breakdowns, it might prove ineffective and expensive due to potentially superfluous maintenance tasks performed on equipment that remains in satisfactory condition.
Both these approaches have been around for years and can be seen the most today. Stochastic statistics and optimization methods often try to guess when machines will fail, but given different cycle times, product mixes, and a variety of other inputs into physical assets, this has now become even more complex and rigorous for manufacturing organizations to solve for.
A new approach is to use artificial intelligence to solve these challenges. It starts with enhancing data collection and management capabilities. When data undergoes consolidation and analysis through AI-enabled signal processing, it can yield a better understanding (extending beyond individual machines) that encompasses the broader network of interconnected assets.
By leveraging the combined insights from humans, sensors, and systems, businesses can employ AI to analyze data and generate maintenance suggestions. These recommendations can be ranked in priority, making more effective use of human resource allocation, so they address the most urgent issues and the equipment most likely to fail.
"Essentially, the AI acts as an ever-present maintenance assistant, aiding human decision-making in determining optimal timing and locations for operational focus." -Sokratis Vasilas Share on XOnce the data infrastructure is set up, the next step is then leveraging machine learning capabilities to identify the “what,” “when,” and “how” for the various physical assets across a manufacturing system.
Machine learning algorithms can develop models capable of forecasting equipment failures using historical data. These models improve in accuracy over time by continuously learning from fresh data inputs. This continuous improvement results in more precise and timely predictions, resulting in more effective per-emptive maintenance, better schedules, less downtime due to failure, and better business outcomes overall.
Recommended: Where AI Really Matters in the Supply Chain
Another critical area of predictive maintenance is fault detection and diagnostics. Many operators often spend time and money trying to pinpoint the root cause of a process that may be negatively affecting a component or even output of their system. Embedding AI within diagnostics and through the analysis of data patterns, AI algorithms can precisely identify the underlying causes of potential problems, enabling focused maintenance interventions. This not only minimizes downtime but also ensures that maintenance efforts are directed towards resolving the particular issues impacting equipment performance.
Virtual Assistants and Copilots
How many hours do you find yourself as a project manager spending doing tedious tasks that bring little to no value within a project?
AI can dramatically reduce this “busywork.” Virtual project managers or assistants can take on these daily administrative tasks. These tasks can include: writing emails, compiling meeting summaries, updating project schedules, validating tasks, and tracking member performance.
According to Gartner, by 2030, the implementation of AI will result in the elimination of 80% of the current workload associated with traditional project management functions such as data collection, tracking, and reporting.
These project assistants will manage fundamental project management duties, thus freeing project teams from the myriad of mundane, low-value tasks, and enabling them to tackle more challenging, strategic, and valuable tasks.
This is why we can expect a strong merging of existing and emerging technologies for human-computer interaction in project management. We can also expect the traditional role of a project manager overseeing a Project Management Office (PMO) and its personnel to give way to intelligent project assistants, such as chatbots.
Industrial Manufacturing Needs to Embrace AI to Enable Successful Transformation
The industrial manufacturing sector is undergoing a profound transformation, driven by challenges from geopolitics, technology, pandemics, and climate change. Despite significant investments, the evidence is incontrovertible; we need the support of advanced technologies to help deliver long term and sustainable transformations.
How AI Can Maximize Value in Project Delivery in Advanced Manufacturing… Share on XManufacturers face the urgent need to adopt innovative approaches to navigate these complexities effectively. Digital twins offer a risk-free environment for product development, enabling simulations that inform design adjustments before physical production. Machine learning is revolutionizing project management by providing tailored insights and optimizing project setups based on specific parameters, ultimately enhancing success rates. Predictive quality and maintenance leverage AI to collect and analyze data, enabling proactive interventions and optimizing resource allocation. The integration of virtual assistants into project management promises to streamline administrative tasks, freeing up human resources for more strategic endeavors.
Manufacturers must embrace AI and advanced technologies to drive operational efficiency, maximize value delivery, and stay competitive in this ever-evolving landscape.
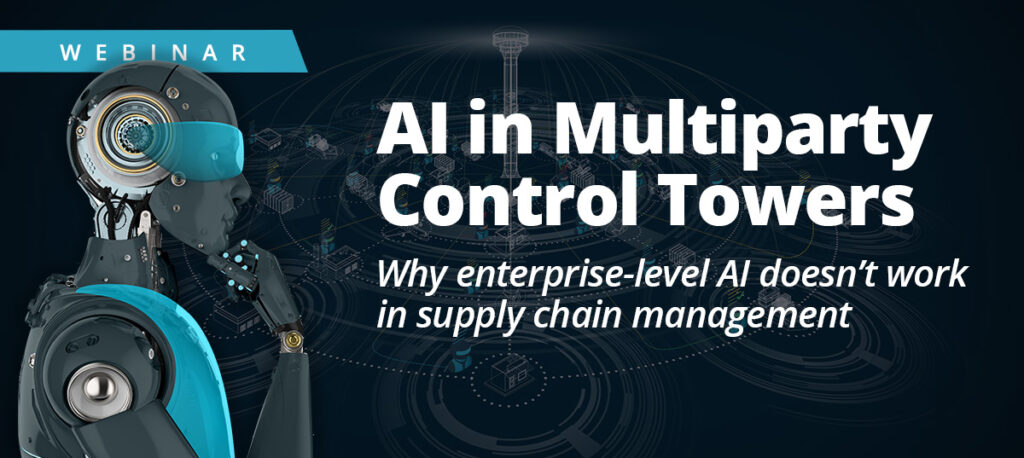