This post has already been read 12862 times!
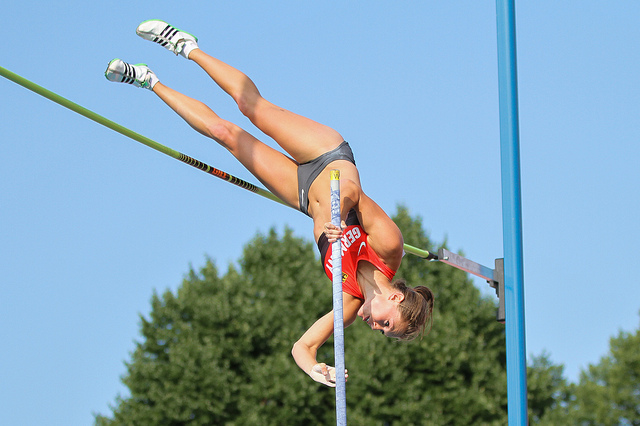
Note: An upcoming webcast will feature this topic as well as an actual demand sensing project between one of the world’s largest CPG companies at the world’s largest retailer. Reserve your seat here!
This is part four in a series on demand sensing. Part one is here. Part two is here. Part 3 is here.
Information theory shows us that increasing mathematical precision to model a “perfect fit” will reach a point where further sophistication of time-series analysis no longer is able to improve upon forecast accuracy. As a proof point, industry surveys show that despite highly tuned models, forecast error remains a significant challenge for most corporations. And the high forecast error rate isn’t limited to slow movers which can experience error rates of over 60%. Even high-volume products with well-understood seasonality patterns continue to experience high near-term forecast error rates of over 40% while using sophisticated time-series methods for demand planning. These forecast techniques are best at predicting much longer horizons and aggregate levels of insights than what is required in today’s consumer driven world.
Just looking at typical real time events helps us understand why many techniques can’t possibly work in the near term. Extreme examples might include a financial downturn or recovery, fluctuations in energy prices, a medical outbreak of some type, regional factors, or some type of a natural disaster. However there are many everyday occurrences that will affect demand like changing weather patterns, such as cold snaps or heat waves, which alter consumer demand from historical patterns. It therefore comes as no surprise that time series models are ill-suited to predicting demand in today’s consumer driven markets.
Demand Sensing vs. Demand Management
Demand sensing is fundamentally different in that it uses a much broader range of demand signals (including current data from the supply chain) and different mathematics to create a more accurate forecast that responds to real-world events such as market shifts, weather changes, natural disasters, consumer buying behavior etc.
Demand Sensing allows companies to incorporate detailed short-term demand data into their forecasts to reduce their forecast error by up to another 50%, increase inventory accuracy by up to 20%, and optimally deploy available inventory whether it’s in transit, shipped from the DC, cross docked, flowed through or shipped direct from manufacturing. Given that retail level forecasts tend to run at an error rate of 40%, this then reduces the error rate to 20% or in other terms gives us an 80% accuracy rate at retail.
By using more frequent and near real-time customer or channel data, daily demand trends are quickly identified, providing advanced warning of problems which will affect customer service levels. Given that the network-based platform has removed the latency between the plan and execution (or in other words what is really happening in the supply chain), issues are elevated quickly, intelligent responses developed, and actions taken, driving an overall immediate response using a single version of “demand” truth.
I’ll discuss this further in future posts. If you’re impatient though, I suggest you read the new white paper, What is Demand Sensing? Much More than a Demand Management Revolution.
- Generative AI: Force Multiplier for Autonomous Supply Chain Management - May 23, 2024
- Top 5 Signs Your Supply Chain is Dysfunctional - August 19, 2022
- Why a Network Model Makes Sense for Automotive Suppliers - July 30, 2019