This post has already been read 18442 times!
How to use generative AI to unlock business value in supply chains
With the emergence of Generative AI the world, and as importantly the stock market, has come to anticipate a huge increase in productivity. Businesses have a tremendous opportunity to capitalize on these technologies not only for supply chain productivity improvements but for overall efficiency, resilience, and agility.
Unfortunately, the market is headed in the wrong direction and, unless it changes, will fail to achieve the productivity gains it is expected to.
Generative AI: Force Multiplier for Autonomous Supply Chain Management – what is really needed to achieve productivity gains in supply chain with generative AI… Share on XThe Evolution of Planning Tools
The current market has tried in the past to solve the supply chain efficiency problems, or seize opportunities, by implementing planning technology to better plan the supply chain. Since I was heavily involved in defining these technologies at the time, I can say that the market achieved significant success with this first advancement of advanced planning solutions. Without this step, supply chains would be far less efficient than what they are today.
The market took a wrong turn when it decided to replace these tools with planning tools embedded in the ERP suite, and as Lora Cecere stated in a recent article, this for the most part proved to be a disaster. It created a whole new implementation, retraining cost, and new process efforts, yet created no additional value and we lost many years of opportunity to improve.
“Embedding planning tools in the ERP suite was a big mistake.”
Greg Brady
Unfortunately, now the market is poised to make its second major mistake by moving to what people are referring to as “modern planning tools”. These tools are however based on the same batch planning memory models of the past so this move will not achieve the promised goals. These systems will provide little to no value around supply chain productivity and it doesn’t matter whether you call it a digital brain or concurrent planning – it will not achieve the goals of significant productivity gains. At best, they will only be able to help in long-term planning.
The Problem with Traditional Planning Tools
So why can’t these planning tools deliver the agility, productivity, and resilience that the market is asking for?
- Batch planning tools move data from execution to the planning model thus creating multiple versions of the truth.
- These multiple versions are based on stale data so not only is planning and execution working on different versions of the truth, but the planners are also depending on information that can be several days or weeks old.
- Since the data is stale the planners are working with lead times that are significantly different from what inventory will actually be available.
- The batch planning systems can only replan the entire supply chain but can not incrementally fix only what is broken. As a result, you’ll never get to autonomous real time systems because the solution would create significant nervousness by redoing the entire plan every time it ran.
For these reasons, this outdated paradigm gave rise to two well-known expressions.
- “Decision Support” implies that you cannot trust the algorithm to make the decision because it cannot be right.
- “I can’t execute the plan” highlights the inability to execute what the plan says if it is leveraging a different version of the truth, data that is days old, and the assumption that inventory is available when in actuality it isn’t. And to resolve it, one must rerun the entire plan even though only a few things are wrong.
Globalization and Outsourcing Exacerbated the Problem
It was bad enough, but it got a lot worse when the world outsourced. Because now the planning tools cannot see the data from manufacturing or raw material or logistics, and yet these tools are attempting to make intelligent supply chain decisions.
“How can planning tools make intelligent decisions when you have multiple versions of the truth for every trading partner, invalid lead times, weeks old data, and no idea of decision impacts? They can’t.”
Greg Brady
How can it, when you have multiple versions of the truth for every ecosystem member, all running on different lead times, looking at data that is weeks old, and making decisions that they have no idea what the impact will be in the wider ecosystem?
As I said, this approach was best in class 20 years ago but can never be used to drive an autonomous and generative (prescriptive) AI system.
What’s Really Needed for Autonomous Generative AI in Supply Chain Management
The market needs to move from decision support technologies and plans that cannot be executed, to autonomous and prescriptive solutions.
Only these solutions can scale to millions of buffer locations addressing problems throughout the day using data from all nodes in the ecosystem that is provided in real time on a single version of the truth.
At least six things are required to make this work:
- The ability to represent, in a multi-tier network, all members of the ecosystem. These members must be able to collaborate around common goals but also have the ability to optimize locally.
- Data needs to be fused together and made available in a single integrated supply chain model in real time and on a single version of the truth for all parties.
- The AI system needs to be able to make decisions continuously as execution issues arise and incrementally replan only the areas that need to be fixed.
- Decisions made by the AI system need to understand the full impact across the supply chain and weigh the cost versus benefit of the decisions.
- The AI system should learn on its own and adjust its lead times, safety stocks, and other parameters based on changes in variability either seen or anticipated.
- Finally, to transition from the old world to this truly modern next generation of technology, the AI should be able to be controlled by setting guardrails and engage the user as an Assistant (Generative AI) but also offer “prescriptions” or recommendations along with the cost benefit analysis.
In sum, we will not get to autonomous supply chain management and realize the benefits by applying AI to the current supply chain technologies.
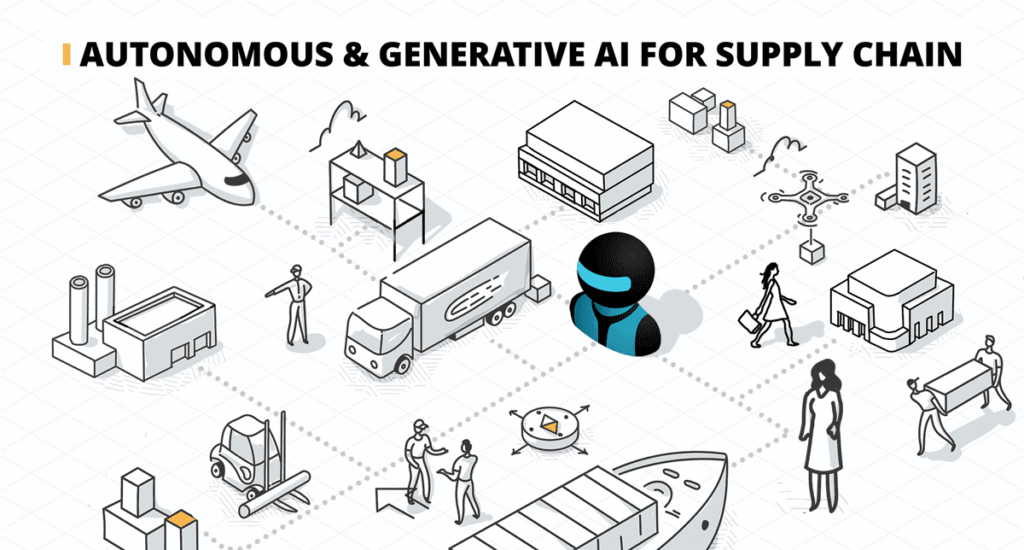
- Generative AI: Force Multiplier for Autonomous Supply Chain Management - May 23, 2024
- Top 5 Signs Your Supply Chain is Dysfunctional - August 19, 2022
- Why a Network Model Makes Sense for Automotive Suppliers - July 30, 2019