This post has already been read 18087 times!
Why effective prescriptive analytics in supply chain requires combined planning and execution
Growing up in Boston MA I participated in many of the chess tournaments held at the Prudential Center. I was always amazed at how well the grand masters played the game. Years later their prowess was on display as some were able to beat early attempts by IBM to defeat them using AI.
The advantage the grand masters had was being able to combine planning and execution. The early computerized capability could sort through large numbers of moves, but was missing the ability to lay in a plan based on rapid pattern recognition, and then adjust that plan in real time, based on the opponent executing the next move. The grand master, on the other hand, essentially combined planning and execution to win the game.
On May 11, 1997, everything changed. IBM’s Deep Blue computer beat the world chess champion.
Today, and from a broader gaming perspective, the computer can now beat the grand master by using ML, combinatorial optimization, neural nets and rapid pattern recognition, through a process which combines planning and execution.
Supply Chain Planning & Execution
Think about how this applies to your supply chain network. You have laid in a plan and are executing to the plan. Then, like a chess opponent, suddenly demand or supply makes an unexpected or unplanned move. You must execute a response which is time-sensitive. Based on this execution response you may need to adjust your plan, given that the network context has changed, just like a grand master adjusting the win-plan based on an unexpected move by their opponent.
The problem, of course, is that your system limitations are significant given that your supply chain network models are not interrelated due to your system silo’s. You can’t really drive an On-Time In-Full (OTIF) outcome of 98% as a single objective because it involves lots of moving parts across multiple systems.
How to Make Artificial Intelligence Work in Your Supply Chain: Disconnects in the supply chain make it impossible to drive optimal outcomes, like achieving OTIF objectives… Click To TweetFor real-world decision-making, most all apps or app-groups need to touch the same supply chain network models. For example, manufacturing, replenishment, warehouse management (WMS), manufacturing planning, multi-echelon inventory planning (MEIO), etc., all need access to some part of the bill of materials (BOM). They are interrelated in the moves you make similar to the pieces on the chess board utilizing the same real estate.
In traditional systems these capabilities reside in their own silos, thus making the right move in real time, and adjusting the plan on that basis, very difficult, because you constantly have to try and update and synchronize copies of data between these systems.
Thus, with these traditional software systems, extending and scaling moves that combine planning and execution across trading partners in the supply chain network becomes nearly impossible. You can’t win.
For example, to solve for an issue related to an OTIF objective, you typically use spreadsheets to combine order, DC, carrier, and logistics information, while adding in information that the systems don’t know (since they are not real time); all while you are trying to execute a real time response.
From a workflow perspective you receive an update from your logistics visibility solution that there is going to be an estimated time of arrival (ETA) problem. From there you are on your own bouncing between systems and stale data. You are operating like the early AI systems that pick the next best decision available without understanding the impacts in other areas, or without connecting dynamically through the entire workflow through to customer delivery.
Your company is probably using AI, most likely on a correlation basis to improve forecasting, eliminate bias, determine inventory policies, or predict disruption due to external vectors like weather. This is good, but it is like the early days of AI at IBM where the grand master could still generate better outcomes than the system.
It is better to combine planning and execution using AI and prescriptive analytics to win the game with your system, which in the supply chain network world is delivering product at the highest customer service levels at the least landed cost.
Using AI to Optimally Solve Supply Chain Problems
Using AI and prescriptive analytics on a causal basis to produce improved outcomes is a much better approach than many of the corporate applications of AI/ML today. As shown in the graphic below the network solution space sort of looks like a lumpy chess board. The objective is to land at the top of the solution space. For example, a low altitude solution would produce 95% in store in stock using 65 days of supply from manufacturing through retail. A solution at the peak which is arrived at by applying a sequence of optimal prescriptions would produce 99% in store in stock using 25 days of supply.
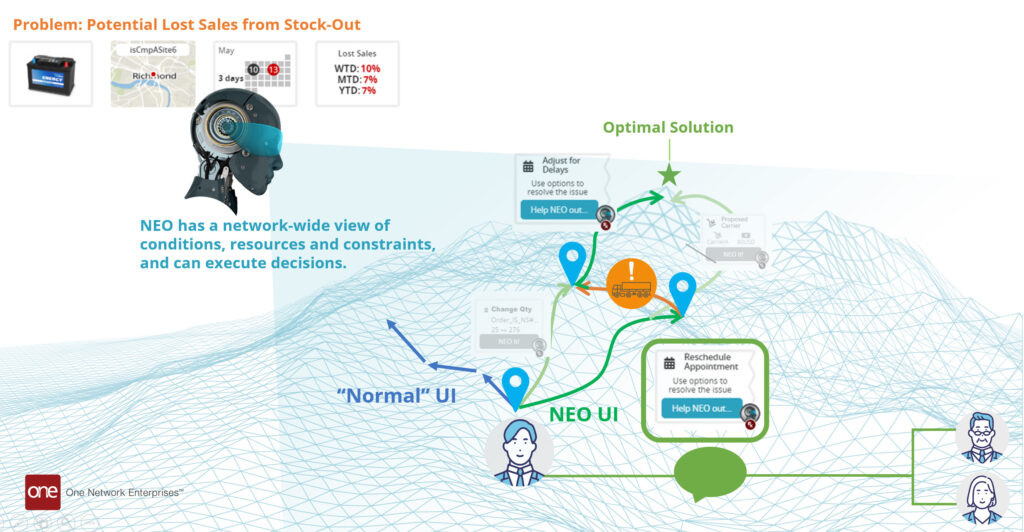
Your typical user as depicted in the lower left, is going to make their next best move depending on the choices in their silo, whether they are trying to solve for orders, logistics, demand, supply etc. If they get lucky they may solve for multiple dimensions. But more likely, given all the parties involved, they run a few spreadsheets, refer to a few predefined scenarios from the planning system, send out a few emails, make a few phone calls… and hope for the best.
By combining planning and execution in real time and leveraging prescriptive analytics you achieve the scenario on the right side. This is where the user interacts with the system through a series of prescriptions to ultimately arrive at the solution peak, which drives the highest customer service levels at the least landed cost.
The example showcases the ability to execute the next move while planning the downstream moves based on generating the winning outcome. It also depicts the ability to adjust the downstream plan based on an unexpected event, such as the ETA prediction of a late arrival.
"Leveraging AI and prescriptive analytics on a single platform that combines supply chain planning and execution, delivers the best outcomes and value for the customer and all trading partners." -Joe Bellini Click To TweetLeveraging AI and prescriptive analytics in this way generates the best outcomes, but it requires combining planning and execution, along with the ability to remember decision-making patterns for future application.
As we can see, incorporating causal-based AI prescriptions allows you to really take advantage of AI through the combination of planning and execution just like the evolution of AI to generate better outcomes than the grand masters.
Bringing Grand Master-Beating Strategies to the Supply Chain
The way it works is that with each prescription the system updates the downstream plan through to customer delivery using a sequence of prescriptions. If the context changes, similar to a chess opponent making a move that wasn’t expected, the system will adjust its prescriptions just like the grand master would adjust their plan based on what was just executed. The system will remember the sequences that generated the best outcomes and apply those in the future which is the same method applied by the evolved AI systems that beat the grand masters.
Driving real world results and outcomes by applying prescriptive analytics, causal-based ML, combinatorial optimization, and neural networks, requires a real-time network representation of the trading partner ecosystem. This network representation enables the ability to combine planning and execution to drive exceptional value for our customers, their customers, and their suppliers. This is what One Network has achieved with its Digital Supply Chain Network™.
Our current release drives further innovation in the area of machine learning applied to supply chain networks. The release includes an enhanced underlying framework with ML plug points (“bring your own intelligence”), as well as enhancements to the NEO learning agents, such as the carrier recommender agent and ETA predictor agent. The goal of the predictor is to take advantage of the real-time single version of the truth and network of networks platform to provide best-in-class prediction accuracy, with the ability to take action in a prescriptive workflow when ETA issues arise to resolve for orders, logistics, materials, capacity, labor or any asset related problem.
This release also marks the introduction of NEO Hyperbots. These are user-defined intelligent bots which automatically execute smart prescriptions on behalf of the user. This is taking this unique prescription framework to levels that generate ever increasing value for customers. Smart prescriptions are getting embedded into more and more services and workbenches across the platform.
Run Your Supply Chain Better Than a Grand Master
In conclusion, seamless planning and execution combined with prescriptive analytics drives tremendous capabilities, as we saw in the evolution of the IBM chess solution. Applying this capability across a supply chain network to generate value is greatly enhanced by ML-based prescriptive analytics to leverage the gold mine of data available for decision-making across your enterprise, your customers, and your suppliers.
By the way, the above example is a real case study, where a CPG Manufacturer was able to deploy dynamic workflow across planning and execution resulting in improved in-store in-stock while at the same time reducing inventories in the network by over 50%.
What kind of results can AI in the supply chain deliver? From 95% in-store in-stock service level with 65 days of supply, to 99% in-store in-stock with only 25 days of supply. Huge inventory reduction with higher service levels. Click To TweetIf you want to generate real business value by leveraging AI/ML and prescriptive analytics, then One Network’s NEO Platform, which combines planning and execution leveraging real time data across your supply chain network, is your answer. Just ask the grand masters who were eventually beaten by a similar AI/ML capability.
- 8 Actionable Supply Chain Trends for 2023 - January 19, 2023
- Constraint-Based Supply Planning & Execution - November 21, 2022
- Supply Chain Planning Control Tower - September 29, 2022