This post has already been read 15000 times!
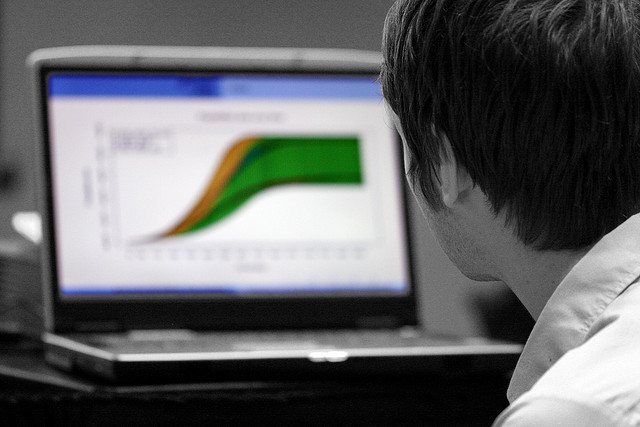
Note: An upcoming webcast will feature this topic as well as an actual demand sensing project between one of the world’s largest CPG companies at the world’s largest retailer. Reserve your seat here!
This is part five in a series on demand sensing. Part one is here. Part two is here. Part 3 is here. Part 4 is here.
Demand Sensing can import demand data on an hourly rather than a batch or daily basis, immediately sensing demand signal changes as compared to a detailed statistical demand pattern, and then evaluates the statistical significance of the change. The automated routines analyse partial period actual demand and execute short-term forecast adjustments using a combination of heuristics, probabilistic pattern recognition, and predictive analytics. Advanced statistical analytics identify and rapidly react to replenishment issues or sudden changes in customer demand.
While being driven directly by high frequency POS/consumer data at the SKU/shelf/location level will generate the highest forecast error reductions, the mathematics around pattern recognition and predictive analytics will also drive significant levels of improvement if applied by other supply network nodes including retail DC’s, manufacturing DC’s, or any integrated suppliers.
Demand Sensing is really a call to action for every company because now the capability exists to manage the entire process from demand signal capture to both short-term and long-term forecasting, resulting in increased sales revenues and decreased inventory costs.
What About The Data?
Breaking the forecast accuracy ceiling requires the inclusion of current demand signals throughout the supply chain as well as the application of new mathematics designed to sort through the masses of data and determine what is truly predictive. There is no shortage of near real-time data collected from the downstream retailers in the supply network all the way upstream through to the manufacturers and their suppliers.
However take care not to misinterpret the data. For example, many of today’s forecasting systems will skip data from days where there were inventory shortages on the shelf! From a demand sensing perspective, this could be the most important data set since we need to predict what consumer demand would have been on the days the inventory was not available on the shelf. Without this more accurate prediction of the actual daily demand we will certainly make poor replenishment decisions, creating inefficiencies upstream which reflect themselves in any planned inventory allocations.
The improved data usage also drives value upstream into manufacturing. According to a McKinsey & Company report, manufacturers can improve their demand forecasting and supply planning by the improved use of their own data. But as we’ve seen in other domains, far more value can be unlocked when companies are able to integrate data from other sources including data from retailers, such as promotion data, launch data, and inventory data. By taking into account data from across the value chain (potentially through collaborative supply network planning and execution), manufacturers can smooth fluctuating order patterns. The benefits of doing so will ripple through the value chain, helping manufacturers to use cash more effectively and to deliver a higher level of service.
- Generative AI: Force Multiplier for Autonomous Supply Chain Management - May 23, 2024
- Top 5 Signs Your Supply Chain is Dysfunctional - August 19, 2022
- Why a Network Model Makes Sense for Automotive Suppliers - July 30, 2019